Designing a new CLARIN Resource Family for semantic change research
A guest post from Professor Barbara McGillivray from the Department of Digital Humanities at King's College, London, introducing a new set of resources and a forthcoming online seminar.
That words change their meanings over time is a fact of language that we all observe in our everyday life. Think of the word snowflake. In addition to the original meaning related to snow, as reported by the Oxford English Dictionary, towards the end of the last century snowflake started being used to refer to people with a “unique personality and potential” and then later on to people who are seen as “overly sensitive or easily offended”. Many of these changes in meaning are the result of cultural and societal shifts, scientific or technological innovations. Sometimes, new concepts like “subscribing to someone’s social media account” are in need of a name and this name is found by adapting existing words, like follow.
Research on this type of change, called “semantic change”, has occupied linguists and lexicographers for a long time. Historical and etymological dictionaries are the go-to places when it comes to tracing the evolution of words and historical linguists have looked into whether there are any regularities across languages in the way words change their meanings. But the interest in semantic change does not stop with linguistics. People from other backgrounds have studied semantic change as a way to better understand how concepts changed in time and what this tells us about our society.
In recent years researchers in natural language processing have taken on this challenge: get computers to help us find semantic change. In simple words, this type of research has created new algorithms that, given some words, can “predict” whether their meaning changed. As an illustration of this, Figure 1 shows the output of Lea Frermann and Mirella Lapata’s algorithm, a topic model trained on a diachronic English corpus spanning 1700-2010. The algorithm is described in their article “A Bayesian Model of Diachronic Meaning Change” (https://aclanthology.org/Q16-1003.pdf). In the output, each bar corresponds to the proportion of each sense for a given time interval, and each sense is referred to via its 10 most probable words. For example, the sense of power as ‘energy’ (violet bars in Figure 1) emerged in the mid-19th century and is correctly detected as a new sense by the algorithm.
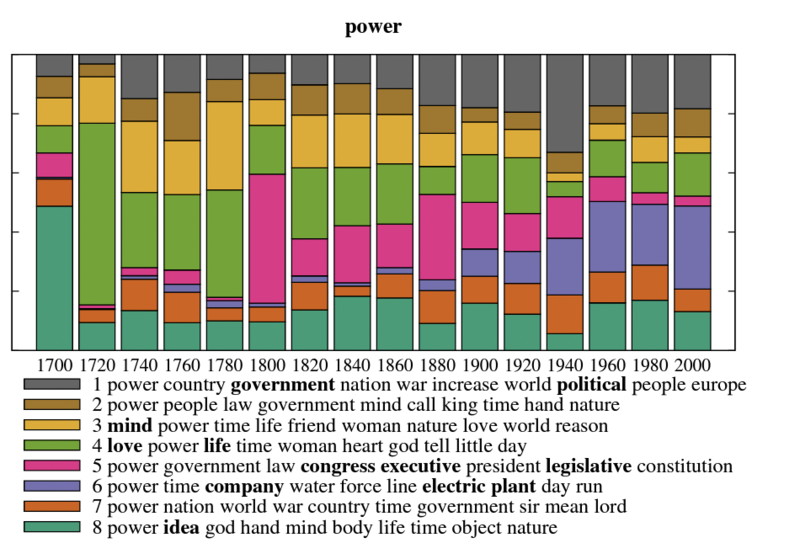
Figure 1: Example output of a semantic change detection algorithm that predicts the distribution of the senses of the English word power between 1700 and 2010. This image is taken from Figure 4 of the article by Lea Frermann and Mirella Lapata “A Bayesian Model of Diachronic Meaning Change” published in the Transactions of the Association for Computational Linguistics, 4:31–45 in 2016 (https://aclanthology.org/Q16-1003.pdf ).
In lexical semantics, researchers have also worked on annotating texts to mark the meaning of words. This requires a detailed analysis of each textual passage to interpret the meaning of a word of interest (“target word”) in its context. For example, in their ancient Greek annotated dataset, published in Figshare in 2019 (https://doi.org/10.6084/m9.figshare.7882940.v1), Alessandro Vatri and Viivi Lähteenoja annotated three words in ancient Greek texts, an example is in Figure 2.
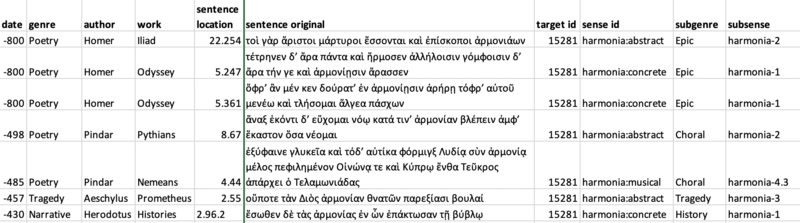
Figure 2: Part of the annotation of the ancient Greek word harmonia which means ‘fastening’, ‘agreement’, or ‘musical scale, melody’ . This annotation is from Vatri, Alessandro and Lähteenoja, Viivi (2019). Ancient Greek semantic annotation datasets. Figshare. https://doi.org/10.6084/m9.figshare.7882940.v1 . We can see the date, genre, subgenre, author and title of the work, the location of the annotated sentence, its text, the token id of the target word in that sentence, its sense and subsense. For example, in the second row we can see that harmonia has been annotated with the concrete meaning (‘fastening’).
Researchers have also designed models that represent semantic change information contained in lexical resources like dictionaries as “linked data” to make it more interoperable. The example in Figure 3 shows the case of the early English wordgurl(the predecessor of the present day English word girl), whose sense ‘a child of either sex; a young person’, according to the Oxford English Dictionary, is recorded in texts from ca. 1300 onwards. Figure 3 associates a life span or temporal extent to each entity.
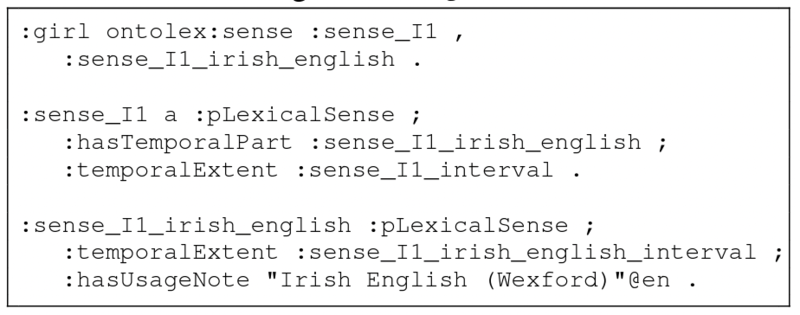
Figure 3: Example of a representation of the first sense ‘a child of either sex; a young person’ of the English word gurl in the Resource Description Framework model proposed by Khan, Fahad (2020). Representing Temporal Information in Lexical Linked Data Resources. In Proceedings of the 7th Workshop on Linked Data in Linguistics (LDL-2020), pages 15–22, Marseille, France . European Language Resources Association (https://aclanthology.org/2020.ldl-1.3 ). This representation models the diachronic lexical information contained in the Oxford English Dictionary, which records that this sense of the word gurl is limited, still today, to a dialect of Irish English.
All these resources and tools are not very easy to find and connect to each other, making it hard for us to advance research in semantic change. Many are available in separate CLARIN Resource Families (CRFs), for example the one dedicated to historical dictionarie or that for manually annotated corpora.
For the CLARIN-funded project “A new CLARIN Resource Family for lexical semantic change research”, Paola Marongiu, Fahad Khan and I are designing a new CRF that brings together tools and resources needed to support semantic change research.
The new CRF, represented schematically in Figure 4, will connect various types of resources and tools: datasets with annotation on words’ meanings in context, word embeddings trained from diachronic corpora, algorithms and tools for automatic semantic change detection, lexical resources and dictionaries.
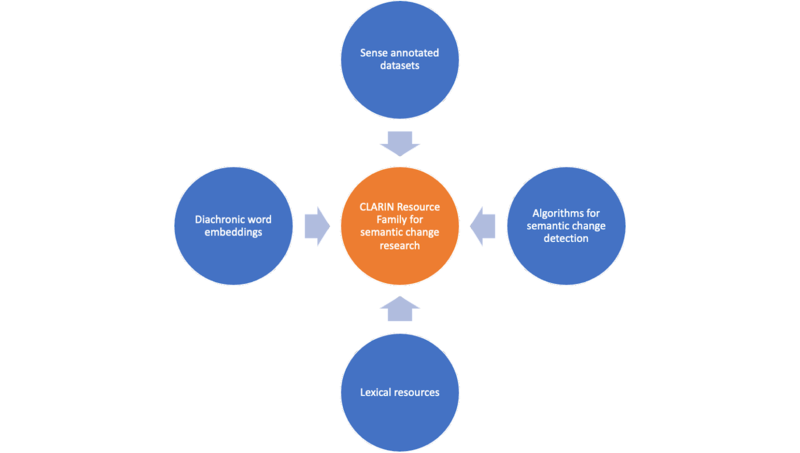
Figure 4: Schematic representation of our proposed CRF, showing how the different resources and tools will be connected.
This CRF will make existing tools and CRFs more discoverable. It will also support multilingual research on semantic change, and more broadly, the study of language as a carrier of cultural content and information. Given the critical role of semantic change research for many humanities and social sciences disciplines, this new CRF will also contribute to strengthening CLARIN’s role to support research in this area. Collecting multilingual language resources together, the CRF will also help advance algorithms for semantic change detection, and language technology research more broadly.
On 5 July 2023 there will be an online CLARIN Café to present our groundwork for the creation of the CRF. As our main focus has been on historical languages (particularly Latin), this CLARIN Café is an opportunity to get input from the broader CLARIN community working on other languages. The event will be accompanied by a tutorial showing how the CRF can be best used for semantic change research and all materials will be available from the CLARIN Café webpage.